🥇 Gemini 2.5 Takes #1, 🖼️ GPT-4o Masters Images, 🩺 Cancer AI >99% Accurate
PLUS: DeepSeek's New Speed 🚀 | Claude's Thinking Trick 🤔 | Apple's $1B Bet 💰
👋 This week in AI
This steady march of AI progress continues - The leading AI labs are updating their models and proving out that scaling laws hold. New image capabilities take the internet by storm and scientific breakthroughs are a weekly occurrence.
We’re living in a time reminiscent of science fiction - The convergence of AI, humanoid robotics and VR means we’re entering a new era.
🎵 Podcast
Don’t feel like reading? Listen to the podcast instead.
Gemini 2.5 Pro: The Top Model Across Multiple Domains
Google has introduced Gemini 2.5, a new AI model family featuring built-in reasoning abilities. The initial release, Gemini 2.5 Pro Experimental, currently ranks #1 on the LMArena leaderboard, reflecting strong user preference.
The model exhibits advanced coding capabilities, scoring 63.8% on SWE-Bench Verified (Claude 3.7 still tops the leaderboard for coding), and excels in reasoning tasks, leading benchmarks like GPQA.
It launches with a 1 million token context window, enabling analysis of extensive data, with plans to increase this to 2 million tokens. Gemini 2.5 Pro is accessible now in Google AI Studio and via the Gemini app for Advanced subscribers.
Why it Matters
This model's integrated reasoning enables more sophisticated analysis and decision-making directly within the AI.
Coupled with the very large context window (1M tokens, expanding to 2M), it allows the model to process and synthesise information from extensive sources like entire code repositories or research collections.
This combination is key for developing more autonomous AI agents capable of handling complex, multi-step tasks involving vast amounts of data, potentially streamlining intricate workflows in coding and analysis. It marks a progression towards AI systems that can manage greater complexity more inherently.
📝 Read Google's blog on the release
ChatGPT's Image Upgrade: GPT-4o Brings Precision & Creative Freedom
OpenAI has integrated image generation directly into its GPT-4o model, accessible via ChatGPT. This treats images as part of the model's multimodal understanding, enabling more precise text rendering within visuals and improved contextual awareness compared to separate systems.
The upgrade excels at producing images containing clear text, such as menus or diagrams, and can handle prompts with 10-20 distinct objects. Users can also refine images using natural language conversation, maintaining consistency across iterations.
This capability replaces DALL-E 3 as the default image generator for most ChatGPT users and is available in Sora, with Enterprise, Edu, and API access following soon.
Why it Matters
This native integration makes image generation a more practical tool for visual communication. The model's improved capabilities facilitate the creation of functional visuals like infographics directly within chat workflows. Conversational editing aids iterative design.
Ironically, while the announcement focuses on practical applications, social media has seen widespread use of GPT-4o to replicate artistic styles, notably creating images reminiscent of Studio Ghibli's aesthetic – a style associated with Hayao Miyazaki, who has previously expressed strong concerns about AI's role in art, viewing it as an "insult to life itself."
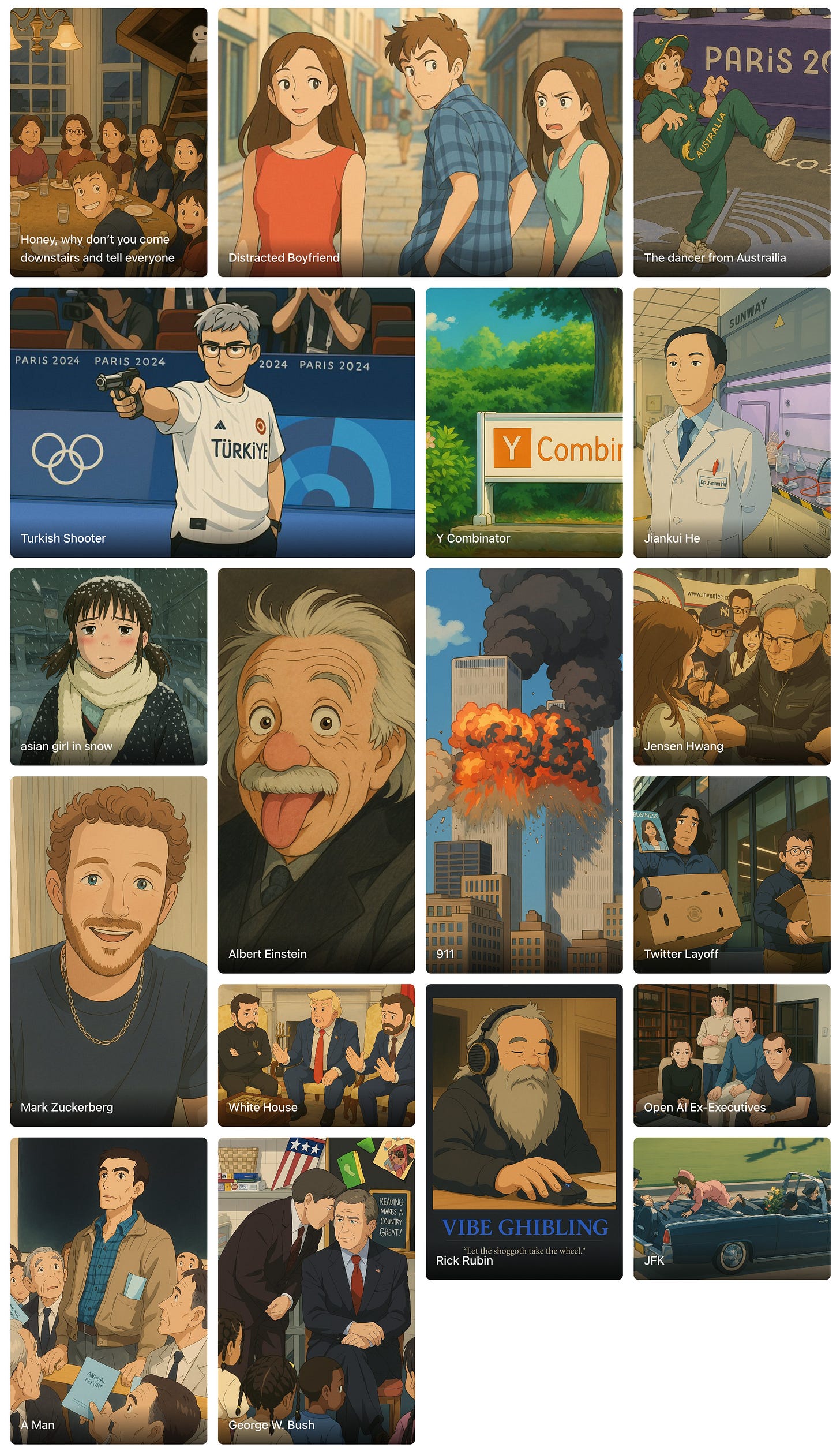
Such stylistic adaptations reflect OpenAI's explicit shift towards greater user creative freedom, granting users increased control over the generated output as highlighted by CEO Sam Altman.
𝕏 Prominent tech images rendered in the style of Studio Ghibli
DeepSeek V3-0324 Unleashed: 685B Power, Desktop Speed
DeepSeek has released DeepSeek-V3-0324, an open-source Mixture-of-Experts (MoE) model with 685 billion total parameters, activating an efficient 37 billion per token. Available on Hugging Face under the MIT License, it demonstrates enhanced reasoning, coding, and translation capabilities.
Key upgrades include improved multi-turn interactive rewriting, better front-end code generation (boosting LiveCodeBench score by 10 points to 49.2), and more accurate function calling with JSON output support. Performance gains are shown across benchmarks like MMLU-Pro (81.2, +5.3) and notably AIME (59.4, +19.8).
Why it Matters
The model offers notable performance increases in complex reasoning and coding tasks, as evidenced by substantial benchmark improvements.
Its efficient MoE architecture, coupled with demonstrated speed on consumer hardware like Mac Studio (20 tokens/second), makes advanced AI capabilities potentially accessible without requiring extensive specialised computing resources.
For developers, enhancements in code generation quality and usability, alongside better function calling, provide more practical tools. This combination of high performance and optimised efficiency could foster wider adoption and experimentation with large-scale AI models.
New AI Boosts Cancer Detection Accuracy Beyond 99%
Researchers have developed an AI model, ECgMLP, designed to detect cancer from microscopic tissue images. Developed by a collaboration including Daffodil International University and Australian universities, the model achieved 99.26% accuracy in identifying endometrial cancer.
This level of accuracy markedly exceeds reported current human diagnostic rates (78-81%) for this cancer type. ECgMLP also demonstrated high accuracy in tests on other cancers, including colorectal (98.57%), breast (98.20%), and oral cancer (97.34%), using specialised attention mechanisms to analyse images.
Why it Matters
The high accuracy shown by ECgMLP suggests a potential for improving the precision of endometrial cancer detection compared to current methods. Its strong performance across multiple cancer types indicates the underlying methodology could be adaptable.
Such AI models offer potential as robust tools to assist clinicians, enhancing decision-making processes for faster and more accurate cancer diagnoses, ultimately aiding better patient outcomes through earlier detection.
Claude's "Think" Tool: Explicit Reasoning Boosts Reliability
Anthropic has detailed the "think" tool, a technique enhancing Claude AI's complex problem-solving. This isn't a separate piece of software but a conceptual prompting strategy.
It leverages the way large language models generate responses step-by-step (autoregressively). By defining a "think" option alongside other tools, developers instruct Claude that it can explicitly generate its internal reasoning process as text before deciding on the next action or final output.
Essentially, when Claude encounters a complex point (e.g., after receiving data from another tool), it can choose to use the "think" tool. This prompts it to generate text like "Let me analyse these results against the policy..." or "Step 1 is done, now I need to check X...". This generated 'thought' becomes part of the ongoing context, guiding the model's subsequent text generation more effectively, much like how writing down steps helps a human solve a complex problem.
Benchmarks show this improves reliability significantly, with up to 54% relative gains in task success.
Why it Matters
The effectiveness of the "think" tool stems from making the AI's internal reasoning process explicit within its own generated context.
By forcing the model to articulate its thought process, analysis, or plan at crucial moments, it creates a richer, more focused basis for its subsequent actions or responses.
This structured self-correction mechanism is particularly beneficial in complex, multi-step scenarios where maintaining context, adhering to rules, or processing intermediate results correctly is vital.
It leads to more reliable and consistent behaviour in applications like AI agents navigating intricate workflows or policy-driven customer interactions, as the model is actively guided by its own explicit reasoning steps.
📝 Blog post by Anthropic on the tool
Apple's $1B Nvidia AI Server Buy Follows Siri Setbacks and Leadership Reshuffle
Apple is reportedly making a substantial investment in generative AI infrastructure, ordering approximately $1 billion worth of advanced Nvidia servers. The purchase is said to include around 250 Nvidia GB300 NVL72 systems, costing between $3.7 million and $4 million each.
Dell Technologies and Super Micro Computer are named as key partners assisting Apple in building this large-scale AI server cluster.
This move follows reported internal challenges, including setbacks in integrating AI features and delays pushing back the planned modernisation of Siri, potentially until 2027.
Why it Matters
This reported hardware investment signals Apple's move to acquire the necessary computing power for advanced AI development, seemingly in response to internal hurdles. Difficulties integrating AI, slower-than-expected progress, and the delayed Siri revamp highlight the challenges Apple faces.
Securing this large-scale Nvidia infrastructure provides a foundation to potentially accelerate development and address these issues, aiming to enhance its competitive position in the rapidly evolving AI landscape.
📝 Apple's leadership reshuffle (paywalled)
📰 Article by Investors on the infra purchase